AI in healthcare and the opportunities for innovation
Reading time 16 mins
Key Points
- AI in healthcare is transforming medical practices at a fast pace and improving preventative care
- Machine and deep learning, when combined with computer vision, have robust capabilities for cancer and tumour detection as well as for creating 3D model simulations of internal organs for more accurate diagnosis
- The combination of AI and IoT created Ambient Intelligence technologies capable of minimising patient waiting time, automating emergency care, and helping the elderly to live more independently
- Genetic testing and AI is creating opportunities for precision medicine and personalised treatments
- Challenges to overcome include the systemic bias of AI software, data protection, ethics and privacy
Looking to revolutionise healthcare experiences with technology? We're here to design health tech solutions that can redefine care provision. Make contact now to find out more.
Ben Mazur
Managing Director
I hope you enjoy reading this post.
If you would like us to develop your next product for you, click here
Humans, by design, are hardwired to resist change – probably because it’s perceived as a threat and triggers our survival instinct. Technologies with clear-cut benefits, such as environmental monitoring tools to predict natural disasters, are wholeheartedly embraced. Others, such as artificial intelligence (AI) or robotics that can make us redundant are understandably met with more resistance. But with few things being more fundamental to survival than our health, the benefits of AI in healthcare (i.e. fast, accurate, and efficient medical analysis or diagnosis), especially during the pandemic, drastically lowered that resistance.
This, in turn, is creating more opportunities for innovation, especially regarding:
- Computer Vision
- Machine Learning
- Deep Learning
- Natural Language Processing
- Ambient Intelligence
AI is the ‘ability of a computer program to perform tasks or reasoning processes usually associated with intelligence in a human being’. A YouGov survey commissioned by the innovation foundation Nesta found that more than two-thirds (68%) of UK workers risk losing their jobs within the next decade. While fears of being made redundant by AI are therefore well founded, this also presents a unique opportunity for skills development (e.g. software programmers and engineers) and innovation. Considering that the global market for AI in healthcare is expected to reach USD 208.2 billion by 2030, resistance would be futile.
Machine learning, computer vision, deep learning, and AI
One of AI’s most significant advantages in healthcare is diagnostics and its focus on preventative medicine. When combined with machine learning, computer vision, and deep learning, the capabilities of AI have become more robust and are transforming healthcare at an even faster pace.
Machine learning (ML) and deep learning (DL) are both types of AI [1]. The former trains on smaller datasets and needs more human intervention to correct and learn (e.g. Spotify algorithms that make personalised recommendations based on what you like). DL is a subset of machine learning but uses artificial neural networks inspired by the human brain to create more complex correlations. DL requires longer training and larger amounts of data to learn from its environment and past mistakes autonomously (e.g. self-driving cars that know that a stop sign covered in snow is still a stop sign).
Computer vision (CV) allows computers to see, analyse, and understand visual data. Visual data plays a crucial role in identifying anomalies and diagnosing diseases. If we rely on our senses exclusively, not only can we fail to pick up on details imperceptible to the human eye that signal a problem, but also more susceptible to human error (e.g. fatigue, stress). CV uses advanced algorithms to assist with image processing and reads it in real-time – simultaneously identifying specific signs of abnormality.
At present, CV and DL are being successfully used [2] to:
- Help with the detection of brain tumours
- Create interactive 3D model visualisations of internal organs and tissues (medical image analysis) to enable more accurate diagnosis
- Improve medical student training by using surgical simulations
- Monitor and estimate the amount of blood lost during surgery, which helps surgeons determine how much a patient will need during/after surgery
- Remotely monitor patients, e.g. detect if a patient recovering at home has fallen
Computer vision and skin cancer detection
Many of us would never think of going to a dermatologist to have a small mole examined – but skin cancers such as melanoma – which can spread to other organs in the body – grow quickly. Melanoma skin cancer incidence rates in the UK are projected to increase by 7% by 2035 – an average of 32 cases per 100,000 people [3]. The good news is that skin cancer is highly curable when detected and treated early.
This has prompted developers to create smartphone apps for skin cancer detection that help users to monitor moles and lesions. The idea is that anyone can download an app, take a picture of a suspicious-looking lesion and allow the app to trawl through the database and determine if the image has potentially cancerous features (e.g. uneven borders) that require further testing.
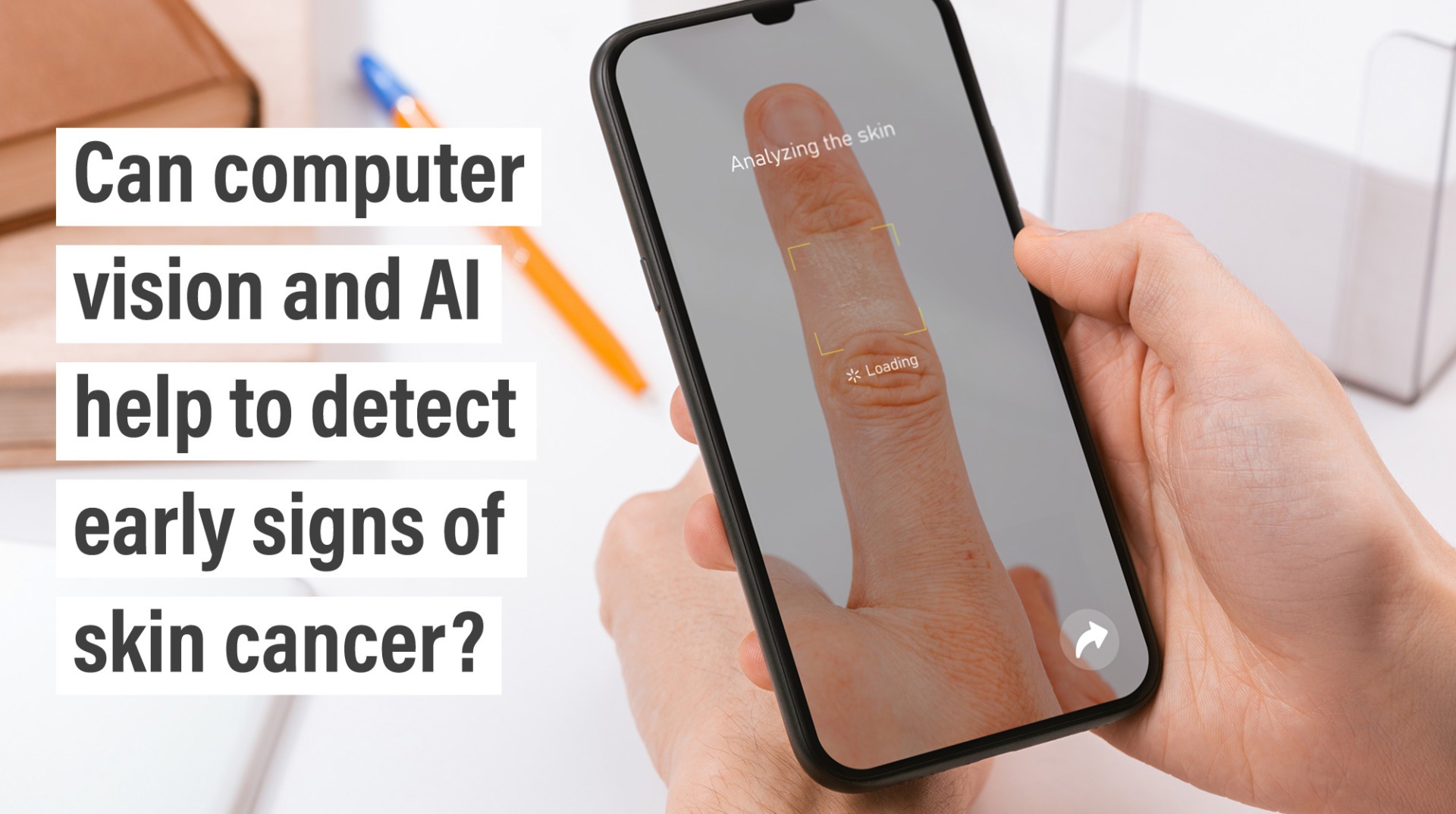
The challenge with skin cancer apps is that they haven’t been proven effective in self-monitoring or skin cancer detection [4]. A systematic review to evaluate the algorithms used on five popular apps found that test accuracy was variable and unreliable. In addition, each study had a high risk of bias (i.e. the darker the skin tone, the higher the likelihood that the apps misclassified the legions).
The opportunity for skin cancer apps is to address existing apps’ flaws (e.g. skin tone bias). How can deep learning be used to make these apps more accurate and capable of complex correlations? Considering that DL requires large datasets to learn from past mistakes, the input needed (i.e. pictures of lesions/moles taken by users and their correct classification) would have to be shared with third parties (i.e. software developers). This gets tricky when data protection needs to be regulated at the same time.
Using genetic testing and machine learning for precision treatment
The challenge with Western or traditional medicine is that it’s designed to treat large groups of people with the same disease. Although these medications take factors such as age, gender, and weight into consideration; most treatments are based on what’s likely to work for everyone with a similar illness [5]. However, not everyone responds to medicines in the same way, and one is never sure what side effects they could have. Finding the right medication that works for you for chronic or complex illnesses could require a fair amount of trial and error.
The opportunity for personalised or precision treatment is that doctors can examine your genetic profile, lifestyle, and environment to deliver treatments specifically tailored for you. While there is still much to be done in this field to make it a widespread practice, genetic testing combined with AI and internet technologies has dramatically improved the medical understanding of diseases, treatments, and preventative practices:
- Using genetic testing and machine learning to predict whether a recipient will accept/reject an organ transplant
- Developing more effective cancer treatments. Genetic tests indicate our susceptibility to certain diseases, and with existing conditions (e.g. tumours), they can identify biomarkers that enable the use of targeted treatments.
- Pharmacogenomics. Understanding how we will respond to a particular medication based on our genome, e.g. trastuzumab (a breast cancer drug) is used to treat women whose tumours have a protein called HER2
Genetic testing certainly has advantages – especially in helping us identify a predisposition towards certain diseases. However, tests can be expensive, and people in remote areas might not have access to testing facilities. Direct-to-consumer genetic testing kits have their limitations but are cost-effective, accessible, and could also provide some of the answers we might be looking for (e.g. genetic markers for diabetes). When combined with IoT-based medical devices, the impact of AI in healthcare is transformational.
What happened when the internet of things (IoT) met AI?
They got married and had a baby.
What started as a concept by tech companies is now a reality, reshaping healthcare even further. Ambient Intelligence (AmI) is the offspring created by the marriage between IoT and AI. Internet-based devices and sensors are placed in the user’s surroundings (e.g. home, office, hospital room) and collect contextual data, which AI uses to predict what the user will need. Siri or Alexa are common examples.
The opportunities for AmI in healthcare are numerous [6]. By using contactless sensors and wearable devices embedded in hospital settings to collect data (e.g. body temperature, audio and images) that ML algorithms can analyse and interpret, hospitals can:
- Minimise patient waiting time: Preliminary tests (e.g. examining a patient’s vitals) and diagnostics reports can be conducted autonomously
- Automated emergency care support: Critically ill patients need continuous monitoring, and many hospitals are understaffed. AmI helps to fill the gaps (e.g. sensors that monitor temperature humidity, blood pressure, and respiration) by collecting critical data autonomously and updating doctors continuously – even when doctors/patients are in remote locations
- Ambient Assisted Living (AAL) technology supports a growing population of senior citizens to live independently. AAL in homes, for example, allows for remote monitoring and brings the point of care (POC) directly to the user
- Thermal computer vision cameras combined with IoT and AI can identify surfaces with infectious viruses that need decontamination. This combination could also help monitor crowded areas and identify people carrying a contagious disease
The challenges with AmI in healthcare are two-fold: ethical and technological. The continuous collection of large amounts of data in healthcare settings (e.g. waiting rooms) where patients/visitors may not be aware that said data is being collected or haven’t consented to it presents numerous ethical challenges. Particularly regarding data protection and privacy, data management, systemic bias and fairness [7]. Then there are the social implications, and the impact that being continuously monitored has on the patient-physician relationship (e.g. trust and confidentiality), as well as the the conduct of physicians and patients themselves. Could AmI systems increase clinicians’ exposure to liability or reduce their sense of responsibility?
Natural Language Processing: Is AI in healthcare multilingual?
Natural language processing (NLP) is the driving force behind AI. This is what helps computers to understand the way that we write and speak – which is a difficult task. It involves interpreting vast amounts of unstructured data and interpreting linguistic styles that are unique to an individual and change according to popular usage, e.g. slang and trending verbal expressions. Typical uses include chatbots and virtual assistants. In a clinical setting, NLP and machine learning help to [8]:
- Search, analyse and interpret patient datasets
- Make sense of the high volumes of unstructured patient data (e.g. doctor’s notes, lab reports) that are entered into electronic health records systems (EHRs) daily
- Translate unstructured data into a useable format that’s easier for algorithms to extract and made machine-readable
- Discover previously missed or improperly coded patient conditions
- Streamline administration (e.g. extract pertinent info from health records and chart notes), thereby freeing up the time that physicians and staff spend on paperwork
The challenges of NLP in healthcare relate to its linguistic limitations. Although there are over 7000 languages spoken globally, most NLP processes only use seven: English, Chinese, Urdu, Farsi, Arabic, French, and Spanish. Improved health is a global issue; thus, the technologies used to drive AI in healthcare should be easily accessible – regardless of the language one speaks. Similarly, not all physicians who speak the same language will do so in the same way. Variations in medical terminology and uncommon acronyms can confuse NLP coding algorithms and result in inaccuracies.
The opportunities for NLP in healthcare are in addressing its challenges. As much as 80% of all healthcare data is said to be unstructured [9], but NLP has the power to harness that and transform it into a format that can be employed in numerous ways – without needing a human to read and edit it first. This begs the question:
- Can NLP be combined with AmI devices (e.g. microphone) to start the process of structuring patient data from the initial doctor-patient consultation? This would eliminate the need for NLP software to make clinical notes machine readable at a later stage and ensure that structure is built into every phase of the patient’s healthcare journey
- Can NLP understand nuance and context? For example, an insurance company assessing a disability claim will read medical records differently than a surgeon diagnosing an illness. The words are the same, but the context matters
- How can NLP be developed to reduce bias? [10] The accuracy of NLP software and AI, in general, is only as good as the data received. Take, for example, a Toronto-based company that developed a speech cognitive assessment tool to detect Alzheimer’s disease. Only to realise after publishing their research that the software was only accurate for English speakers of a specific Canadian dialect
Do the opportunities for improved healthcare using AI outweigh the challenges?
The challenges that AI in healthcare need to overcome are significant but manageable. Not only do these technologies have the ability to democratise healthcare and make it more efficient, but the focus that it puts on preventative care results in beneficial outcomes for everyone involved.
Trust will play a significant role in how readily these technologies are accepted and deployed – oversight and regulation will be crucial. In our next series of posts, we’ll take a closer look at how medical devices and technologies – including software and AI – are regulated. What impact will proposed reforms have on the engineers and developers who create them? How will this affect the people – medical practitioners and patients alike – who use them?
If you’re as curious as we are, signup for our newsletter, and we’ll keep you posted.
Comments
- Deep Learning vs. Machine Learning: Beginner’s Guide (2022, August 11) Coursera. https://www.coursera.org/articles/ai-vs-deep-learning-vs-machine-learning-beginners-guide
- Boesch, G. (2022, August 12). Top 10 Applications Of Deep Learning and Computer Vision In Healthcare. viso.ai. https://viso.ai/applications/computer-vision-in-healthcare/
- Cancer Research UK. (2022, May 31). Melanoma skin cancer statistics. https://www.cancerresearchuk.org/health-professional/cancer-statistics/statistics-by-cancer-type/melanoma-skin-cancer
- Petrou, I., MD. (2020, November 17). Smartphone apps not effective in skin cancer risk. Dermatology Times. https://www.dermatologytimes.com/view/smartphone-apps-not-effective-in-skin-cancer-risk
- How Is Precision Medicine Different From Traditional Medicine? (2018, May 30). WebMD. https://www.webmd.com/cancer/precision-vs-traditional-medicine
- Sanyal, S. (2022, October 17). Top 10 Ambient Intelligence Trends for the Healthcare Industry in 2023. Analytics Insight. https://www.analyticsinsight.net/top-10-ambient-intelligence-trends-for-the-healthcare-industry-in-2023/
- Martinez-Martin, N. (2021, February 1). Ethical issues in using ambient intelligence in health-care settings. The Lancet Digital Health. https://www.thelancet.com/journals/landig/article/PIIS2589-7500(20)30275-2/fulltext
- Natural Language Processing in Healthcare Medical Records. (n.d.). ForeSee Medical. https://www.foreseemed.com/natural-language-processing-in-healthcare
- Safavi, K. (2021, April 26). Unleashing the power of unstructured data. Accenture. https://www.accenture.com/us-en/blogs/insight-driven-health/unleashing-the-power-of-unstructured-data
- Krasniansky, A. (2019, October 29). Understanding Racial Bias in Medical AI Training Data. Bill of Health. https://blog.petrieflom.law.harvard.edu/2019/10/29/understanding-racial-bias-in-medical-ai-training-data/
We love to talk about new ideas
Do you have an idea? Book a consultation with an expert - it's free, it's confidential and there are no obligations.
+44(0)117 329 3420
[email protected]
Ignitec Technology Centre
1 The Powerhouse
Great Park Road
Bradley Stoke
Bristol
BS32 4RU
0 Comments